Does the SmartItem Work?
DATA SIMULATION EVIDENCE
Demonstrating Difficulty Equivalence
Background
In the ebook “SmartItem: Stop Test Fraud, Improve Fairness, & Upgrade the Way You Test,” we discuss how SmartItem technology works, how it solves long-standing and important validity issues (specifically the theft of test content, effective cheating, and testwiseness), and how the SmartItem performs psychometrically.
Also in the book, we learn that randomization is an effective and popular method that controls systematic error and balances out the differences between items, including difficulty, introduced by SmartItem technology. When using randomization in SmartItems, we are not doing things much differently than our current day-to-day practices of creating equivalent or equated forms, or pulling items from a pool with CATs or LOFTs. Indeed, we can all accept a bit of randomness in our exams, especially if it eliminates or reduces major sources of systematic error.
In the research paper “SmartItem: Case Study Evidence,” we learn about how SmartItem technology performs and about the benefits of SmartItem technology through two information technology certification exams. Data presented from these case studies indicate that SmartItem exams have been functioning properly for the purpose of certifying competence in a field.
In parts 1 & 2 of the research papers “SmartItem: Scientific Evidence,” we read about carefully controlled experiments. These experiments show that SmartItem technology performs well according to the statistical criteria used to evaluate item performance. The experiments also prove that tests completely comprised of SmartItems are valid and reliable, at least to a similar degree as traditional tests made up of traditional fixed items.
To expand upon this evidence and provide additional support for the use of the SmartItem, this paper discusses two data simulations. The simulations have two goals:
- To demonstrate the equivalence of difficulty for tests with and without SmartItems.
- To show the effect of SmartItems on predicted cheating.
The Value of Data Simulations
The benefits of data simulations are twofold. First, they are convenient to use. Second, conditions that are difficult to replicate or impossible to obtain in case studies can easily be replicated in data simulations.
The simulation described in this paper assumes that items are pulled randomly from an entire domain (e.g., mathematics skills for an eight-year-old student). This is a case of randomization with no constraints. Therefore, the simulation described uses randomization in a broader way than would be expected if SmartItem technology were used. On a test consisting of SmartItems, randomization is limited by the design and functionality of each individual SmartItem. In this simulation, there are no such constraints.
Figure 1 shows a shape that represents a domain. The dots within the shape represent items. For the simulation described here, the items are selected randomly to serve on a test as illustrated by Figure 2.
Mathematics Content Domain
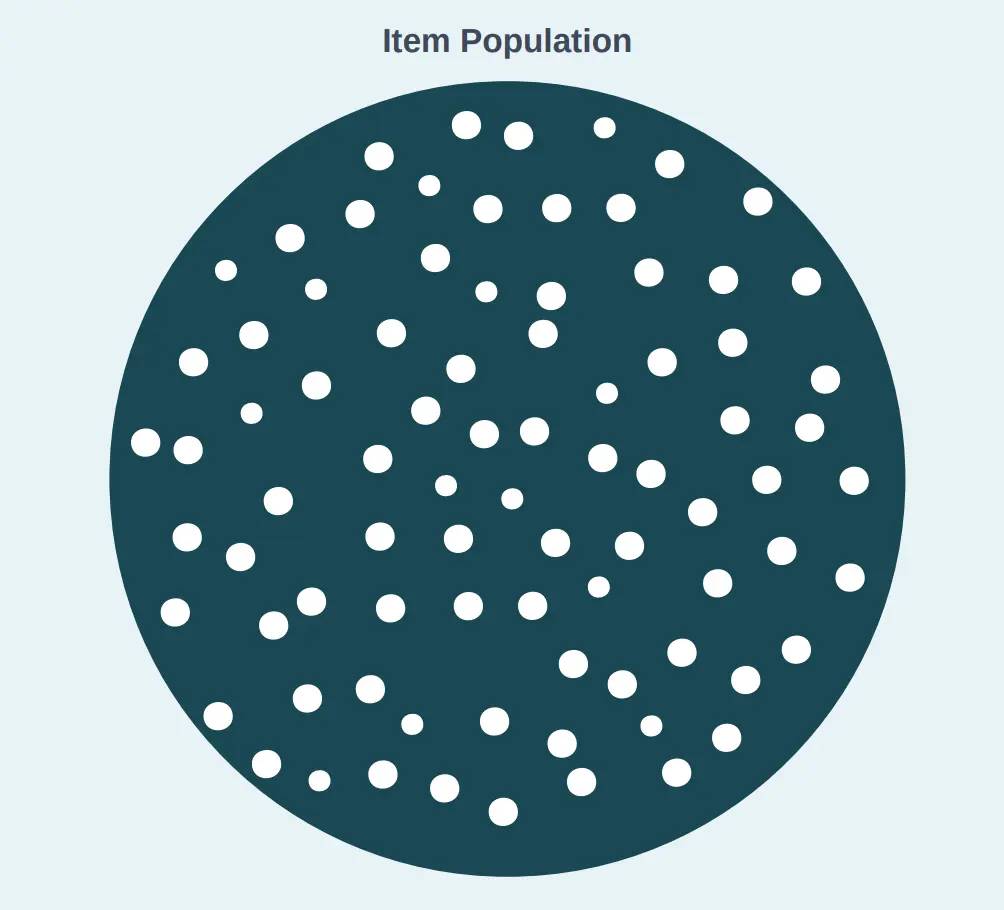
Figure 1
The circle represents a skill domain (for example, an eight-year-old student’s skill with third grade mathematics). Each dot represents an item. Following the example, items are each created to measure any one of the many subskills within the third grade mathematics domain.
Third Grade Mathematics Test
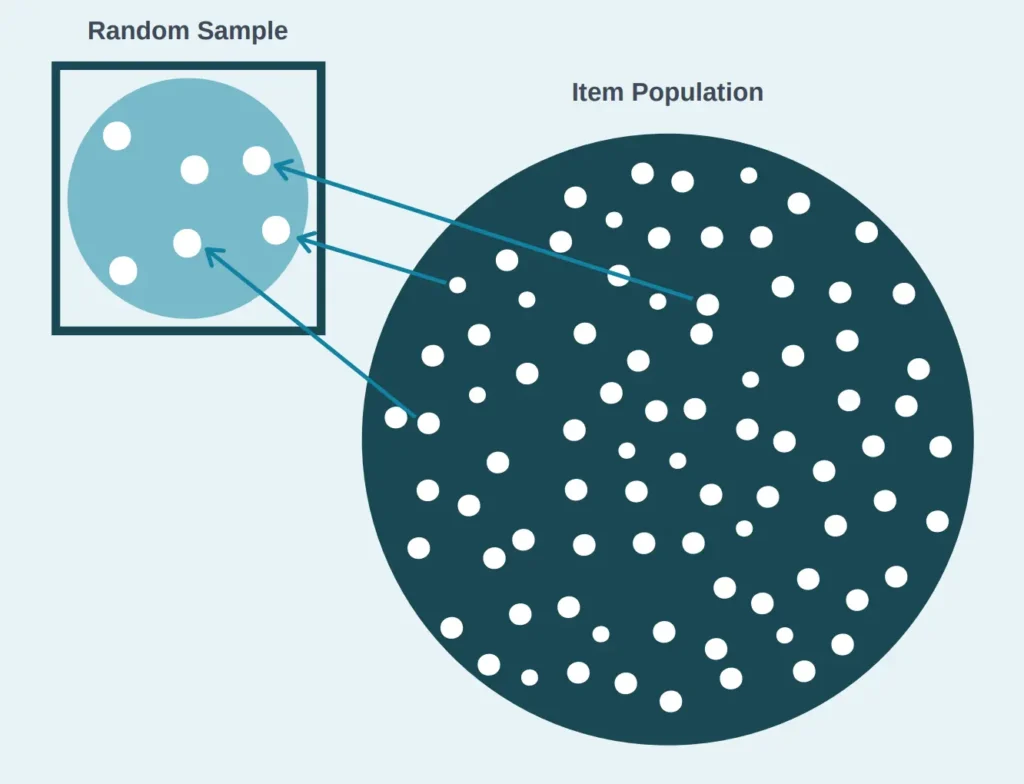
Figure 2
With the purely random selection process, any item representing any skill within the domain can be selected for a test.
Simulation: Broad Randomization from a Content Domain
Theoretically, the randomization of SmartItem content introduces error into a test score—this error is random error. With enough items on a test, the sum of that random error should result in a value (slightly positive or slightly negative) close to zero by the end of the test. This theoretical principle supports this conclusion: each score will be minimally impacted by random error, and can be fairly compared to other scores. In addition, the harmful effects of systematic, secondary, and irrelevant variables such as cheating are prevented from impacting the test scores.
However, the question remains: How many items with randomly rendered content are needed for the random error to achieve a value close to zero for each test taker? Is it the same number of items as a typical test? Is it more? Is it fewer? Can we afford the money and time it would take to administer more items than we have been? The simulation described herein, first reported by Toton et al. (2018), is an initial attempt at answering these questions.
The Simulation:
Data Generation
A bank of 5,000 items was generated using the two-parameter logistic IRT model to describe the distribution of items. The item difficulties were sampled from a normal distribution with a mean b parameter of 0.0 and standard deviation of 1.0.¹ The discrimination parameter was sampled from a normal distribution with a mean a parameter of 1.0 and a standard deviation of 0.05. Tests were then generated that varied in length from 10 to 60 randomly selected items from the item pool.
A set of 100,000 simulated examinees “took” each of the 51 tests. The exams were normally distributed on their theta values with a mean of 0.0 and a standard deviation of 1.0[1]. In addition to these 51 forms of different lengths, each of the 100,000 examinees “took” a fixed-form test comprised of the same 40 items, randomly selected from the same pool of 5,000 items.
Test Differences
It was important to come up with a way to determine if a randomized form (of various lengths) was of different overall difficulty than the 40-item fixed-form test. There are several different methods for determining if a person was given a test that is statistically equivalent or statistically different in difficulty from the fixed test. In this case, we computed the standard error of test difficulty using the calculated p-values (classical test theory calculation; proportion of items answered correctly) for items on the fixed-form test.
This was then used to compute a confidence interval for the test difficulty. The standard error of the mean of test difficulty was computed as the standard deviation divided by the square root of test length. This calculation resulted in a standard error of the mean of .028.
Using the standard error of the mean and applying a 95% confidence interval around the mean of the fixed form, we see the confidence interval for the mean difficulty of the fixed form ranged from .44 to .55.
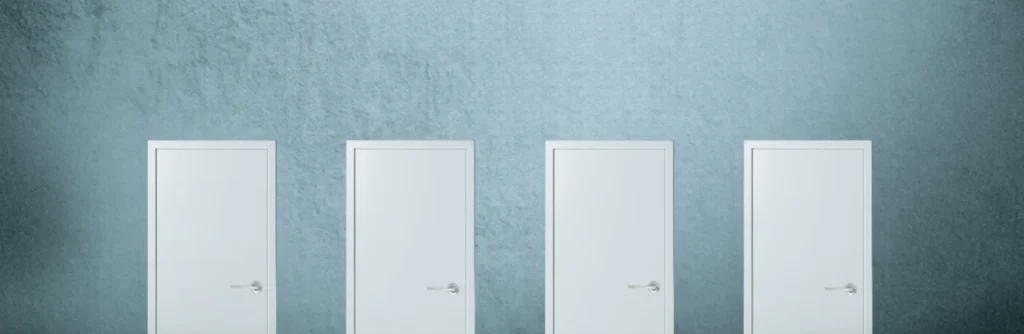
Now, when a simulated examinee received a score (computed as a p value) from a random form, we compared the score of that test to the confidence interval above. If the score (or difficulty) of that random form fell within the confidence interval, we concluded the difficulty of the random form was no different from the difficulty of the fixed form. Otherwise it was treated as non-equivalent.
Finally, we computed the proportion of tests at each test length from 10 to 60 items that were equivalent or different in terms of difficulty, compared to the fixed form. As you can see in Figure 3, when the random test was only 10 items long, .363 (or 36.3%) of simulated test takers had a test that was either harder or easier than the 40-item fixed test.² That means that only 63.7% of the tests would be considered of equivalent difficulty. However, when the random test was increased to 40 items long, only 8.6% of people had a test score that was either harder or easier than the fixed-form test, or 91.4% of them being equivalent in difficulty.
As the number of items increased to 59, the probability of one being a test of different difficulty continued to decrease. For example, for a random form of 50 items, the proportion of examinees who had an equivalently difficult exam was 95.5%. For tests with more than 50 items, more simulated test takers continued to receive fewer tests that were not equivalent in difficulty to the fixed 40-item test. This pattern is shown in Figure 3.
Percentage of Test Takers Receiving Test of Equivalent Difficulty Compared to Fixed Form
10-Item Test: 63.7%
40-Item Test: 91.4%
59-Item Test: 95.5%
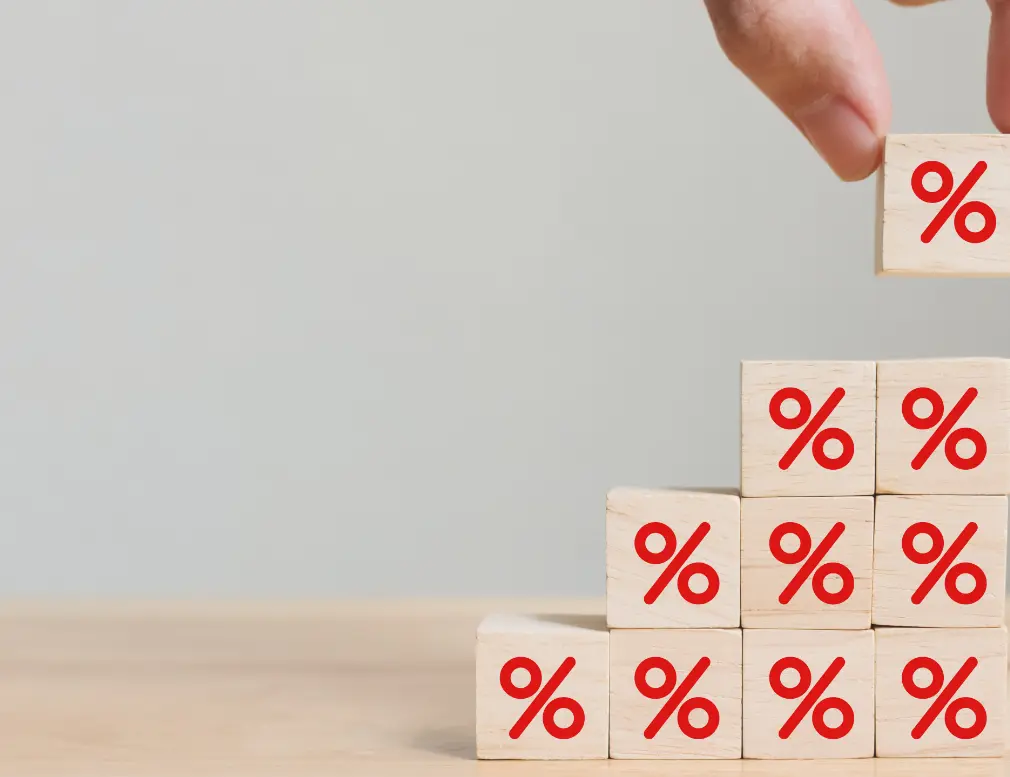
Given the assumptions, values, and methods of the simulation, it seems the random error introduced by items with broadly random content on tests (38 to 59 items) will result in roughly equivalent or score-comparable tests. (Remember, the randomization produced in these simulations is greater than what would be produced by SmartItem technology.)
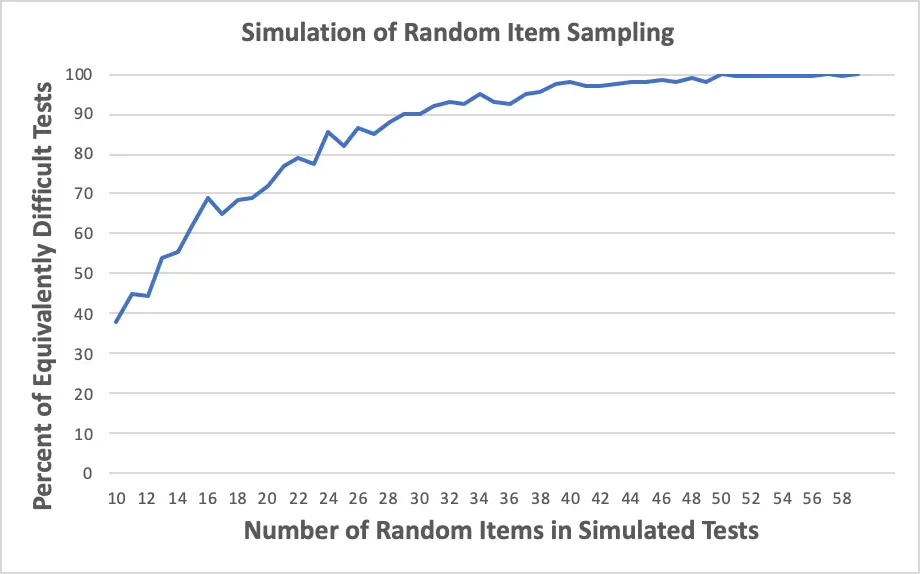
Figure 3
The results from Simulation #1.
Conclusion
Simulations are a popular method for approximating real testing conditions. The simulation described in this paper can be extended by:
- Simulating the randomization of many individual SmartItems on the same test, and
- Repeating the simulation, but varying the length of the fixed-length form and changing the distributions of examinee capability and/or item difficulty/discrimination.
The results of this simulation encourage the use of SmartItem technology when the forms are of sufficient length. Considering that high-stakes tests used throughout the world for many different purposes (e.g., education, certification, etc.) are usually made up of more than 40 items, the randomness on tests will have little to no impact on the difficulty of the test scores.
Footnotes:
- The values for the mean and standard deviation of the ability (or theta) of the examinee sample distribution are common for data simulations.
- If a test has a mean difficulty that is determined to lie outside of the confidence interval, the test could be easier or more difficult.
References:
Toton, S., Williams, T., Foster, C., Foster, D. F., & Foster Green, A. (2018). SmartItems and Their Surprising Effects on Test Security. Paper presented at the Conference on Test Security, Park City, Utah.
Curious If Scorpion Is The Right Platform For You?
Tell us a little about your organization’s needs and request your free demo today!